Artificial Intelligence: An Epistemological Critique
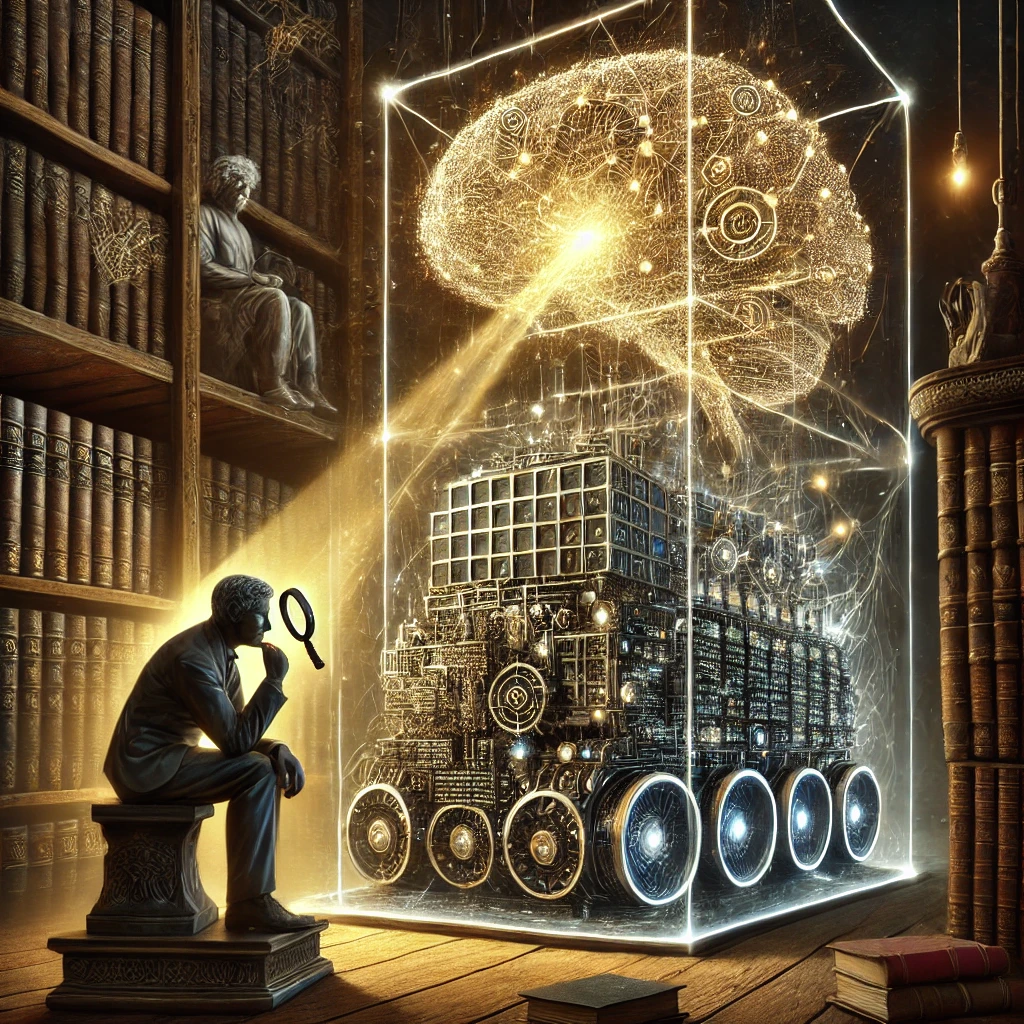
Epistemology, the branch of philosophy concerned with the nature and limits of knowledge, provides a valuable framework for critiquing artificial intelligence (AI). As AI rapidly develops and promises to revolutionize numerous fields, fundamental epistemological questions remain unresolved. Can AI truly be considered a producer of knowledge? What biases and limitations are inherent in using data and algorithms to generate knowledge? Here are some critical reflections on AI through the lens of epistemology.
1. The Nature of Knowledge Generated by AI: Knowledge or Mere Correlation?
One of the central epistemological questions is the distinction between knowledge and correlation. Traditionally, scientific knowledge relies on theories that explain why certain phenomena occur, often with an underlying causal dimension. AI, however, primarily functions by detecting correlations in vast datasets.
Thus, when machine learning algorithms identify trends or patterns, it may seem as though new knowledge is being generated. In reality, these results are often limited to statistical correlations without causal explanations. As the saying goes, correlation does not imply causation. This raises a fundamental epistemological question: Can the knowledge produced by AI truly be considered knowledge?
Example: AI systems in medicine can detect associations between symptoms and diseases, but these associations do not always offer explanations. In other words, AI can predict a medical outcome, but it does not understand why the outcome occurs, unlike scientific approaches that seek to establish underlying mechanisms (Miller, 2019).
2. Data Bias: A Distortion of Knowledge?
AI algorithms are trained on existing data, which presents a major epistemological problem: Is the data reliable and representative of reality? Biases present in datasets can be directly transferred into algorithms, affecting the outcomes.
From an epistemological standpoint, this raises the question of the objectivity of knowledge produced by AI. If AI systems reproduce or amplify pre-existing biases, such as racial, gender, or economic biases, can we still consider AI to be producing valid knowledge?
Example: Algorithms used in the criminal justice system have demonstrated racial bias when predicting recidivism. Studies have shown that these algorithms tend to classify individuals from minority groups as more likely to reoffend due to the biased data on which they were trained (O’Neil, 2016). This reveals how epistemic biases can distort the notion of “neutral” knowledge produced by AI.
3. Algorithmic Opacity: Unintelligible Knowledge?
AI systems, especially those that use deep learning algorithms, are often referred to as black boxes. Even the developers of these systems may struggle to explain exactly why and how an AI reached a specific decision or prediction. This algorithmic opacity poses a significant epistemological challenge: Can knowledge be considered valid if it cannot be understood?
In science, a fundamental principle is that explanations must be understandable and verifiable. However, modern AI systems, due to their complexity, often elude human understanding. This leads to a form of “knowledge” that is difficult to challenge and whose validation relies more on trust in the results than on true comprehension.
Example: Algorithms used in the financial sector to make investment decisions sometimes generate unexpected returns. However, those responsible for the systems are not always able to explain why the AI recommended certain actions, limiting the transparency and epistemic validation of this financial “knowledge” (Pasquale, 2015).
4. The Status of AI: An Epistemic Agent?
Another crucial question revolves around AI’s status as an epistemic agent. Can AI be considered a knowledge-producing agent in the same way as humans? AI can manipulate information, detect regularities, and even formulate hypotheses, but it lacks consciousness and intentional understanding. This raises the question of whether AI is truly capable of generating knowledge or if it is merely a tool used by humans to interpret data.
For epistemologists, knowledge is more than just the accumulation of information. It involves understanding, intentionality, and critical judgment—qualities that AI, despite its sophistication, cannot achieve. As a machine, AI lacks the capacity for critical reflection, which is central to human knowledge.
Example: In the field of machine translation, AI systems like Google Translate produce impressive results. However, they do not “understand” languages or the cultural contexts in which they operate. Their work is limited to manipulating symbols according to algorithmic rules without any real comprehension of the texts they translate (Floridi, 2020).
Conclusion: AI as an Epistemological Challenge
Artificial intelligence raises significant challenges for modern epistemology. While it promises to transform how we produce and access knowledge, it also presents important limitations in terms of the nature of knowledge, bias, opacity, and the epistemic status of AI itself.
To address these challenges, we must develop a more critical and philosophical approach to AI, taking into account its intrinsic limitations and its implications for the production of human knowledge. Ultimately, AI is not just a technical or technological issue but also a profoundly epistemological one.
References:
- Floridi, L. (2020). The Logic of Information: A Theory of Philosophy as Conceptual Design. Oxford University Press.
- Miller, T. (2019). “Explanation in Artificial Intelligence: Insights from the Social Sciences”. Artificial Intelligence, 267, 1-38.
- O’Neil, C. (2016). Weapons of Math Destruction: How Big Data Increases Inequality and Threatens Democracy. Crown.
- Pasquale, F. (2015). The Black Box Society: The Secret Algorithms That Control Money and Information. Harvard University Press.
0 Comments